AI and Machine Learning for Predictive Healthcare
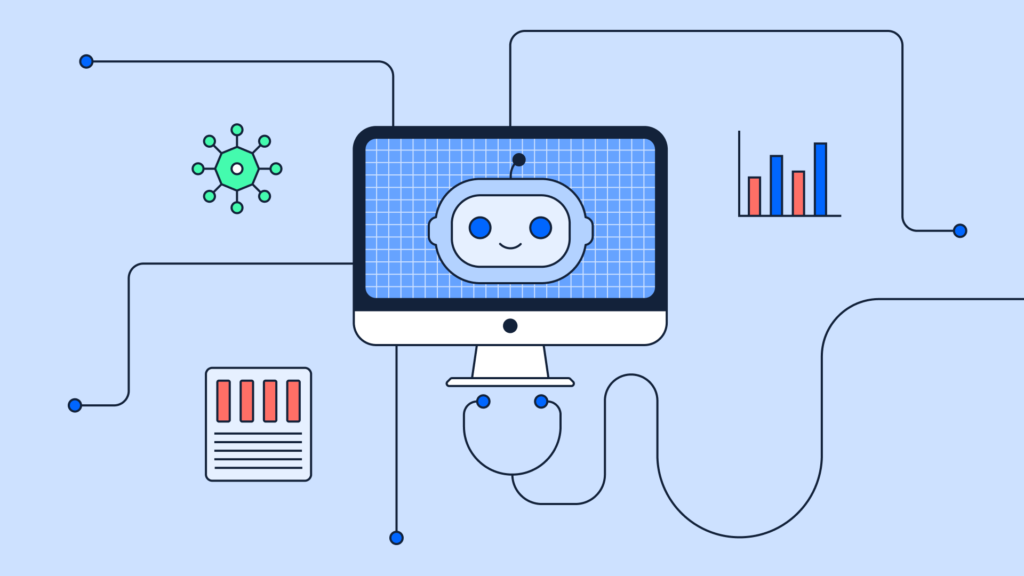
The healthcare industry is on the brink of a paradigm shift, driven by the integration of artificial intelligence (AI) and machine learning (ML). These technologies are redefining how medical professionals predict, diagnose, and treat diseases, with a focus on moving from reactive care to proactive, preventive strategies. Predictive healthcare, powered by AI and ML, aims to identify health risks early, personalize treatments, and optimize outcomes, ultimately transforming patient care and reducing systemic costs.
The Role of AI and ML in Predictive Healthcare
Predictive healthcare leverages vast datasets—from electronic health records (EHRs) and genomic data to wearable device metrics—to forecast health events before they occur. AI and ML algorithms excel at identifying patterns in these datasets that are often imperceptible to humans. By analyzing historical and real-time data, these systems can predict disease onset, anticipate complications, and recommend preventive interventions.
Key Applications of AI/ML in Predictive Care
- Disease Risk Prediction
AI models analyze genetic predispositions, lifestyle factors, and biomarkers to predict risks for conditions like diabetes, cardiovascular diseases, or cancer. For example, ML algorithms can assess retinal scans to predict diabetic retinopathy or use EHR data to flag patients at high risk of heart failure. - Early Diagnosis
Machine learning enhances diagnostic accuracy by processing imaging data (e.g., X-rays, MRIs) and lab results faster and more accurately than traditional methods. Algorithms trained on millions of images can detect early-stage tumors, neurological disorders, or rare diseases with precision rivaling expert clinicians. - Personalized Treatment Plans
AI tailors therapies to individual patients by analyzing their unique genetic makeup, medical history, and response to past treatments. For instance, oncology platforms like IBM Watson for Genomics suggest personalized cancer treatments based on tumor DNA sequencing. - Predicting Hospital Readmissions
Hospitals use ML to identify patients likely to be readmitted within 30 days of discharge. By flagging high-risk individuals, care teams can intervene with follow-up care, reducing costs and improving outcomes. - Drug Development and Clinical Trials
AI accelerates drug discovery by predicting molecular interactions and identifying promising compounds. It also optimizes clinical trial design by selecting ideal participants and forecasting potential side effects, shortening time-to-market for new therapies. - Remote Patient Monitoring
Wearables and IoT devices collect real-time data (e.g., heart rate, blood glucose, sleep patterns) that AI systems analyze to detect anomalies. This enables early warnings for conditions like sepsis or arrhythmias, even in home-based care settings.
Benefits of Predictive Healthcare
- Proactive Care: Shifts focus from treating illnesses to preventing them.
- Cost Reduction: Lowers hospitalizations and emergency visits through early intervention.
- Improved Outcomes: Enhances survival rates and quality of life via timely diagnoses.
- Scalability: Extends expert-level insights to underserved populations through AI tools.
- Efficiency: Automates administrative tasks, freeing clinicians to focus on patient care.
Challenges and Considerations
While AI and ML offer transformative potential, challenges remain:
- Data Privacy: Ensuring patient data security and compliance with regulations like HIPAA and GDPR.
- Bias and Fairness: Mitigating algorithmic biases rooted in unrepresentative training data.
- Integration with Workflows: Adapting legacy healthcare systems to incorporate AI tools seamlessly.
- Regulatory Hurdles: Navigating approvals for AI-driven diagnostics and treatments.
- Clinician Trust: Building confidence in AI recommendations among healthcare providers.
The Future of Predictive Healthcare
Advancements in AI/ML will focus on:
- Explainable AI: Developing transparent models that clarify how decisions are made.
- Federated Learning: Training algorithms across decentralized datasets to preserve privacy.
- Multimodal AI: Combining diverse data types (genomic, imaging, behavioral) for holistic insights.
- Wearable Tech Integration: Expanding real-time health monitoring through smarter devices.
- Collaborative AI: Systems that augment—not replace—clinicians’ expertise.
As these technologies mature, predictive healthcare will become a cornerstone of modern medicine, empowering providers to deliver smarter, safer, and more equitable care.
Stay ahead with ITBusinessNews – Your trusted source for Technology and Business news. Fast & Precise