AI for Climate Change Modeling and Prediction
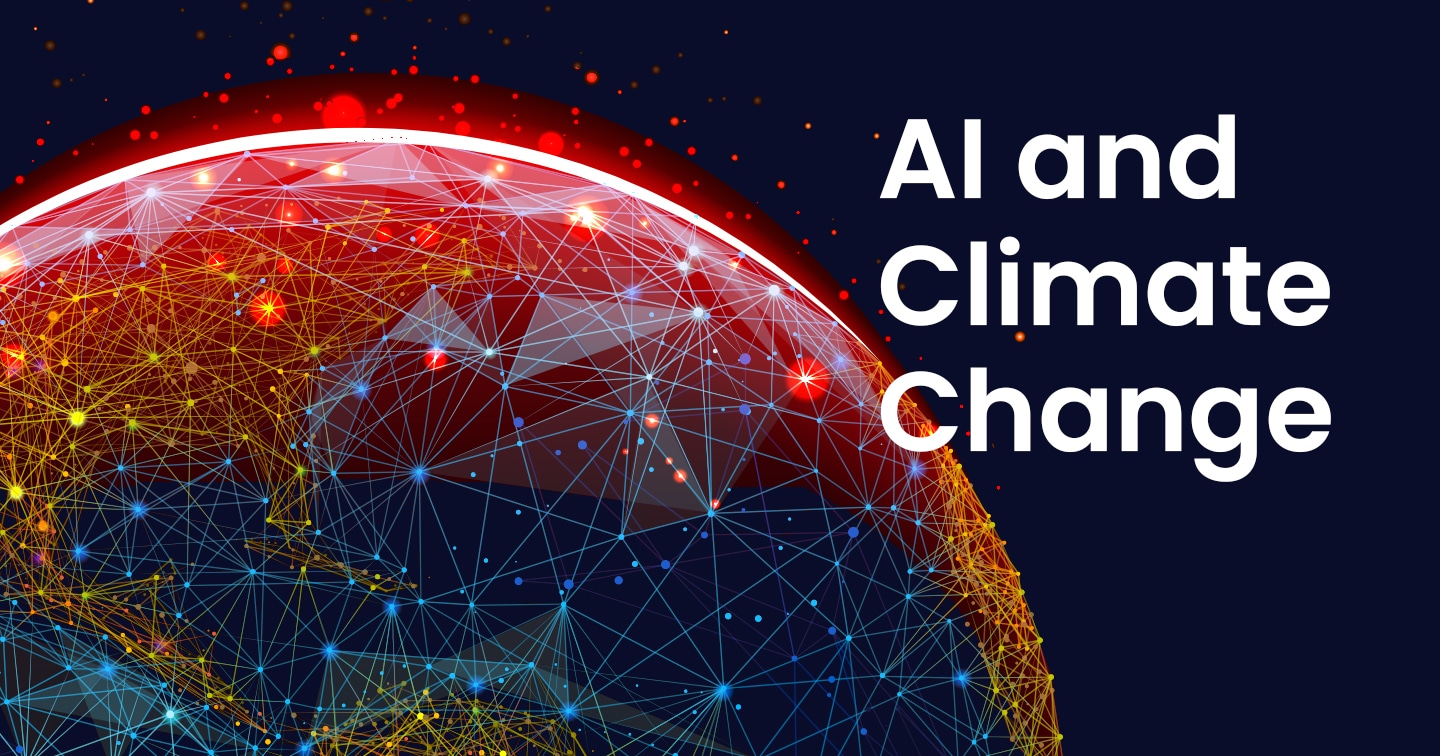
As the effects of climate change become increasingly apparent, the need for accurate and comprehensive climate modeling has never been more urgent. Artificial Intelligence (AI) is emerging as a powerful tool in this domain, offering new ways to predict, understand, and mitigate the impacts of climate change. By leveraging machine learning algorithms, vast datasets, and advanced computational techniques, AI is transforming climate science and enabling more effective responses to this global challenge. This blog explores how AI is being used in climate change modeling and prediction, its advantages, current applications, and the challenges that must be addressed.
Understanding Climate Change Modeling
Climate change modeling involves simulating the Earth’s climate system to predict future changes in temperature, precipitation, sea level, and other critical parameters. These models are essential for understanding how human activities influence the climate and for developing strategies to mitigate and adapt to climate change. Traditional climate models rely on complex mathematical equations to represent the physical processes governing the climate system. However, these models often face limitations in accuracy, spatial resolution, and the ability to incorporate diverse datasets.
AI’s Role in Climate Change Modeling
AI, particularly machine learning (ML), enhances climate modeling by offering tools to analyze vast amounts of data, identify patterns, and make predictions. Here are some key ways AI contributes to climate change modeling:
- Improved Data Analysis AI algorithms excel at processing and analyzing large datasets from diverse sources, including satellite observations, weather stations, and climate sensors. By identifying patterns and correlations in these datasets, AI can enhance the understanding of climate dynamics and improve model accuracy.
- Enhanced Predictive Capabilities Machine learning models can be trained on historical climate data to predict future climate conditions with greater accuracy. These models can learn complex relationships within the data that traditional models might miss, leading to more reliable predictions of temperature, precipitation, and extreme weather events.
- Higher Spatial and Temporal Resolution AI enables climate models to achieve higher spatial and temporal resolution. By downscaling global climate models to regional or local scales, AI helps capture fine-scale climate phenomena that are crucial for impact assessments and decision-making at the local level.
- Integration of Diverse Data Sources AI can integrate data from multiple sources, including atmospheric, oceanic, and terrestrial systems. This holistic approach improves the representation of the climate system and enhances the ability to simulate interactions between different components of the Earth system.
- Real-Time Climate Monitoring AI-driven climate models can provide real-time monitoring and prediction of climate variables. This capability is essential for early warning systems, disaster preparedness, and adaptive management of climate-related risks.
Current Applications of AI in Climate Change Modeling
- Weather Forecasting AI is revolutionizing weather forecasting by enhancing the accuracy and timeliness of predictions. Machine learning algorithms analyze historical weather data and current atmospheric conditions to generate short-term and long-term forecasts, improving the ability to anticipate extreme weather events and mitigate their impacts.
- Climate Impact Assessments AI is used to assess the impacts of climate change on various sectors, including agriculture, water resources, and public health. For example, AI models can predict crop yield changes under different climate scenarios, helping farmers adapt to changing conditions and optimize resource management.
- Carbon Emission Tracking AI helps track and predict carbon emissions by analyzing data from industrial activities, transportation, and land-use changes. This information is crucial for monitoring progress toward emission reduction targets and developing effective climate policies.
- Sea Level Rise Projections AI models enhance the accuracy of sea level rise projections by integrating data on ice sheet dynamics, ocean temperatures, and land subsidence. These projections are vital for coastal planning and risk management in vulnerable regions.
- Biodiversity and Ecosystem Monitoring AI assists in monitoring the impacts of climate change on biodiversity and ecosystems. Machine learning algorithms analyze data from remote sensing and field observations to track changes in species distributions, habitat conditions, and ecosystem health.
Challenges and Considerations
- Data Quality and Availability The accuracy of AI models depends on the quality and availability of data. Inconsistent, incomplete, or biased datasets can lead to inaccurate predictions. Ensuring access to high-quality, comprehensive climate data is essential for the success of AI-driven climate models.
- Model Interpretability AI models, particularly deep learning algorithms, can be complex and difficult to interpret. Understanding the underlying processes and mechanisms driving model predictions is crucial for gaining trust and acceptance among climate scientists and policymakers.
- Computational Resources AI-driven climate modeling requires significant computational resources, including high-performance computing infrastructure and cloud-based platforms. Ensuring the availability and affordability of these resources is a key challenge.
- Integration with Traditional Models Integrating AI models with traditional climate models requires careful consideration of their respective strengths and limitations. Combining the physical understanding of traditional models with the data-driven insights of AI can enhance the overall robustness and reliability of climate predictions.
- Ethical and Social Implications The use of AI in climate modeling raises ethical and social considerations, including data privacy, equity, and the potential for unintended consequences. Addressing these issues requires transparent and inclusive approaches to the development and deployment of AI technologies.
Future Directions
The future of AI in climate change modeling holds immense potential, driven by ongoing advancements in machine learning, data science, and computational capabilities. Key areas of focus include:
- Advancing AI Algorithms Developing more sophisticated and interpretable AI algorithms that can capture complex climate dynamics and improve prediction accuracy is a priority. Innovations in explainable AI (XAI) can enhance the transparency and trustworthiness of AI-driven climate models.
- Expanding Data Integration Integrating diverse and non-traditional data sources, such as social media, IoT sensors, and citizen science initiatives, can provide richer datasets for climate modeling. This expanded data ecosystem can improve the granularity and relevance of climate predictions.
- Fostering Collaboration Collaboration between AI researchers, climate scientists, policymakers, and stakeholders is essential for addressing the multifaceted challenges of climate change. Interdisciplinary partnerships can drive innovation and ensure that AI technologies are aligned with societal needs and priorities.
- Promoting Open Science Promoting open science practices, including data sharing, open-source software, and collaborative research platforms, can accelerate progress in AI-driven climate modeling. Open access to data and tools fosters transparency, reproducibility, and innovation.
- Enhancing Climate Resilience AI-driven climate models can support proactive climate resilience strategies by providing actionable insights for adaptation and mitigation. From urban planning to agricultural management, AI can inform decisions that enhance resilience to climate impacts and reduce vulnerability.
AI is poised to transform climate change modeling and prediction, offering powerful tools to understand and address one of the most pressing challenges of our time. By enhancing the accuracy, resolution, and comprehensiveness of climate models, AI enables more effective strategies for mitigating and adapting to climate change. As technology advances and interdisciplinary collaborations flourish, AI will play an increasingly vital role in shaping a sustainable and resilient future.
Stay ahead with ITBusinessNews – Your trusted source for Technology and Business news. Fast & Precise