AI for Real-Time Traffic Management in Cities
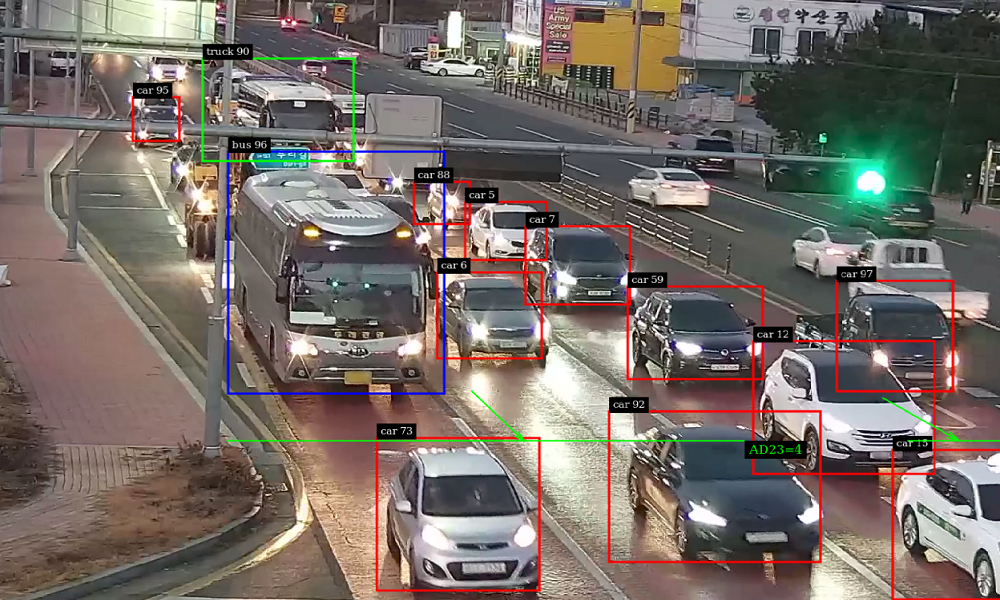
AI is rapidly transforming urban mobility, and one of its most impactful applications is real-time traffic management. In cities worldwide, congestion remains a persistent problem, contributing to pollution, wasted fuel, and lost productivity. AI-driven traffic management systems have emerged as a solution to these challenges, enabling cities to monitor, analyze, and optimize traffic flow in real-time to reduce congestion and enhance commuter experience.
AI-powered traffic management systems collect data from various sources, including traffic cameras, sensors embedded in roads, GPS data from vehicles, and even weather reports. This data is fed into machine learning models that can predict traffic patterns, identify bottlenecks, and detect incidents like accidents or sudden slowdowns. The algorithms continuously analyze these inputs to provide an up-to-date picture of traffic across the city, allowing the system to predict traffic buildups before they occur and suggest alternative routes or adjustments to traffic signals to ease congestion.
Adaptive traffic signal control is one of the key features of AI in traffic management. By adjusting signal timings in real-time based on current traffic conditions, AI systems can significantly reduce wait times at intersections, prevent gridlock, and improve overall traffic flow. For instance, if a large volume of vehicles is detected on one road approaching an intersection, the AI can prioritize a longer green signal to alleviate the traffic quickly. When scaled citywide, these adaptive signals contribute to smoother traffic flow, shorter travel times, and lower emissions from idling vehicles.
AI-based traffic management can also play a role in accident detection and emergency response. By analyzing real-time video feeds and sensor data, AI systems can quickly identify when an accident or road obstruction has occurred and notify authorities for immediate response. Some systems can also predict accident-prone areas by analyzing historical accident data and current conditions, such as weather and traffic volume, enabling cities to take preventive measures. Additionally, these insights can help in routing emergency vehicles more efficiently, ensuring they reach accident sites or hospitals faster.
Another benefit of AI traffic management systems is their integration with public transit. AI can monitor real-time data from buses, trains, and other public transportation modes, optimizing their routes to avoid delays and improving coordination with private traffic. When a public bus is delayed, for example, an AI system can adjust surrounding traffic lights to prioritize its movement, helping it get back on schedule. By making public transit more reliable, these systems can encourage more people to use public transport, which in turn reduces congestion and emissions.
In some cities, AI is also helping to manage road space dynamically by implementing “smart lanes” or prioritizing lanes for high-occupancy vehicles (HOVs) and public transport based on real-time demand. This approach enables city infrastructure to be more flexible and responsive to traffic needs, adapting lane usage patterns throughout the day. For example, during peak hours, more lanes can be designated for outbound traffic in the evening, while the same lanes are adjusted to handle inbound traffic in the morning. Such adaptive measures make better use of existing infrastructure and reduce the need for costly expansions.
Despite its benefits, implementing AI for traffic management presents challenges, particularly in terms of data privacy and infrastructure. Real-time traffic management systems require extensive data collection, which raises concerns about the privacy of drivers and commuters. Addressing these concerns involves implementing strict data privacy regulations, anonymizing collected data, and ensuring that data is used solely for traffic management purposes. Infrastructure compatibility is another challenge; many cities have outdated traffic systems that require costly upgrades to support AI-driven technologies.
The integration of AI in traffic management has enormous potential to shape the future of urban transportation. By making traffic flow more efficient, reducing accidents, and improving public transit, AI-driven systems are paving the way for safer, cleaner, and more efficient cities.
Stay ahead with ITBusinessNews – Your trusted source for Technology and Business news. Fast & Precise