AI in Music Recommendation Systems
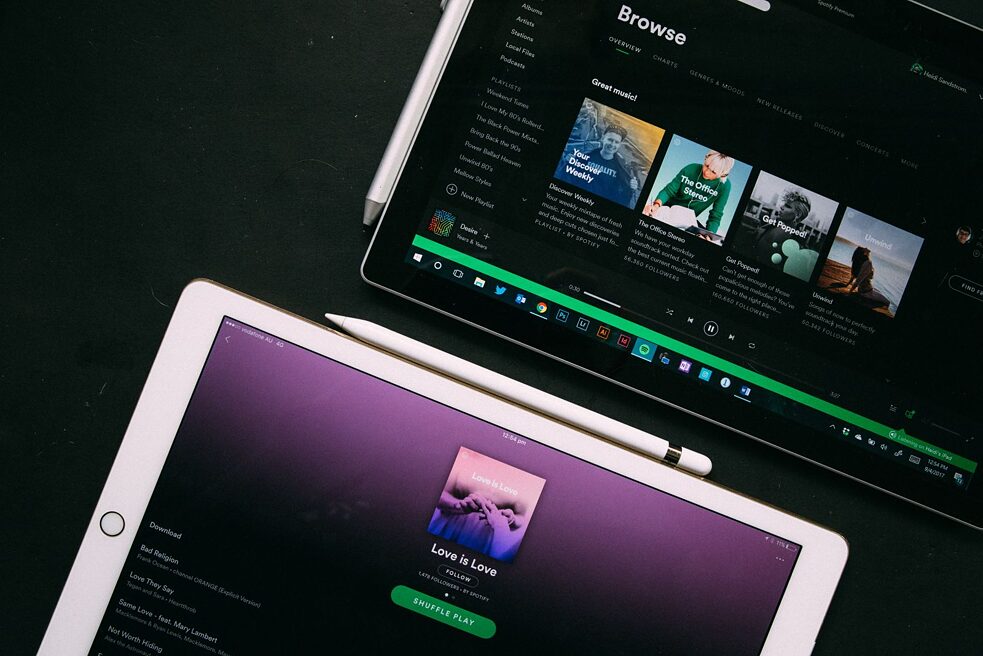
Artificial Intelligence (AI) is fundamentally transforming the way people discover and engage with music. Music recommendation systems, powered by sophisticated AI algorithms, have become an integral part of digital streaming platforms, offering personalized playlists and suggestions that cater to individual tastes. These systems analyze massive amounts of data to predict which songs a listener will enjoy, creating a more engaging and customized user experience.
At the core of AI-driven music recommendation systems is machine learning. These algorithms learn from user behavior, such as listening history, song skips, likes, and search patterns, to understand individual preferences. Over time, this data helps refine the system’s understanding of what kind of music a user gravitates toward, enabling it to suggest songs, artists, and playlists tailored to their tastes. This constant adaptation and learning make the recommendations increasingly accurate as the user interacts with the platform.
Collaborative filtering is one of the primary techniques used by AI in music recommendation. This method groups users with similar listening habits together and recommends songs based on what others with similar tastes are enjoying. For example, if two users consistently like the same songs or genres, the system might recommend tracks to one user that the other has listened to, assuming they would enjoy it too. Collaborative filtering leverages the collective preferences of a vast number of users, allowing for highly accurate recommendations even for new listeners.
Another powerful tool in AI music recommendation is content-based filtering. In this approach, the system analyzes the actual content of songs—such as tempo, rhythm, mood, or instrumentation—and recommends tracks with similar characteristics to those the user already enjoys. This technique is especially useful when recommending new or obscure music, as it doesn’t rely on other users’ preferences but rather on the specific features of the music itself. This combination of machine learning and music analysis ensures that recommendations are based not only on popularity but also on the intrinsic qualities of the songs.
In recent years, deep learning techniques have further enhanced the capabilities of music recommendation systems. Neural networks can analyze more complex patterns in music data, such as identifying how different elements of a song (lyrics, genre, beats) relate to listener preferences. By factoring in both user behavior and the nuanced characteristics of music, these deep learning models generate more sophisticated recommendations that cater to a listener’s evolving tastes.
AI is also being used to analyze the contextual factors that influence music preferences. For instance, music recommendation systems can consider variables such as the time of day, location, or current activity to suggest appropriate songs. If a user frequently listens to upbeat music during workouts or slow instrumental tracks at night, the system can recognize these patterns and adjust its recommendations accordingly. This context-aware recommendation adds an additional layer of personalization, ensuring that users receive music suggestions that fit their specific mood or situation at any given moment.
Another innovation is the use of natural language processing (NLP) to analyze metadata, social media trends, and user-generated content such as reviews or comments. By analyzing how people talk about music online, AI systems can identify emerging trends, popular artists, and hidden gems that might not yet be widely known. This enables streaming platforms to stay ahead of trends and introduce listeners to new music that aligns with their tastes, even before it becomes mainstream.
Despite the many advantages of AI-powered music recommendation systems, challenges remain. One of the primary concerns is the risk of creating a “filter bubble,” where users are only exposed to music that closely matches their established preferences, limiting their exposure to new or diverse genres. This can stifle musical discovery and creativity, as listeners may miss out on music outside of their typical listening patterns. To address this, many platforms incorporate features like “discovery playlists” or “genre explorer” tools that encourage users to explore new music beyond their usual selections.
AI has undoubtedly revolutionized how people discover music, creating personalized experiences that cater to individual tastes. As these systems continue to evolve, the future of music recommendation will likely see even more intelligent and nuanced suggestions that make exploring new music more exciting and immersive.
Stay ahead with ITBusinessNews – Your trusted source for Technology and Business news. Fast & Precise